The challenges encountered in deepwater development have led to the use of increasingly complex subsea systems. Consequently, operators have become more reliant on subsea monitoring equipment and instrumentation to provide field information for understanding production and equipment conditions. Production monitoring is typically the field operator’s main priority, and equipment condition and performance are sometimes overlooked, resulting in equipment failures and long production downtimes because of unplanned maintenance.
Equipment failures often occur without warning and may sometimes, when caused by environmental factors, become inevitable. To determine the cause, field operators sift through vast amounts of distributed data from the subsea control system.
The system downtime can be reduced and system availability can be increased by performing effective equipment diagnosis. With the current low-oil-price environment, subsea operators are looking to maximize returns on investment, and condition-based monitoring offers the possibility of lowering operational expenses while increasing field production.
Condition Monitoring
Condition monitoring is a proactive maintenance strategy combining software and people. Data are used to diagnose changes in the integrity of a system such that corrective action may be planned in a cost-effective manner to increase system availability. Condition monitoring is not a novel technology and is currently used in the aeronautical and automobile industries. The condition monitoring system takes integrity monitoring beyond traditional key performance indicators by utilizing all available data and information from the system and looking for trends of equipment degradation prior to equipment failure.
A condition monitoring system is typically based on the following three-step monitoring process:
- Data Collection: Read-only software to acquire real-time and historical knowledge from the subsea control system. Storing historical data and supporting analysis based on historical trends.
- Analysis: Onshore analysis server applies statistical analysis, signal processing, mathematical modeling, and simulations to ascertain system conditions. Requires experience-based product knowledge and reliability analysis to target known failure modes and performance issues. System may be tuned and updated in order to continuously improve its online diagnostic performance.
- Collaboration and Discussion: Estimation of potential impact on production (availability) is built into the system based on operational and maintenance philosophy. With remote surveillance, multiple onshore and offshore users are able to operate the system on a web-based graphical user interface for monitoring and troubleshooting of the subsea field. To simplify monitoring, each component and associated potential failure mode is designed with its individual user interface.
The status of each component is determined after the processing and analysis of the raw data. The integrity of each component also contributes to the parent component.
Subsea Failure Trends
Based on the field experience of operators, typical offshore and onshore equipment failure trends may be obtained from the reliability data source, OREDA, a database used to collect and exchange reliability data for the improvement of safety, reliability, maintenance effectiveness, and to enhance industry reputation on equipment and plant performance. The database provides subsea equipment performance history for a wide range of equipment from system to component level. This information can be used to predict the availability performance of systems and facilities.
Data from OREDA24 Databank Version 5000.2.1 up to 11 June 2015 was analyzed for the number of failures in various subsystems, including control system, wellhead, subsea tree, template, manifold, and flowline. The control system was identified as the leading contributor of subsea system failures, followed by the wellhead and subsea tree. The condition monitoring system is designed to monitor the subsea conditions with the same order of focus (Fig. 1 above).
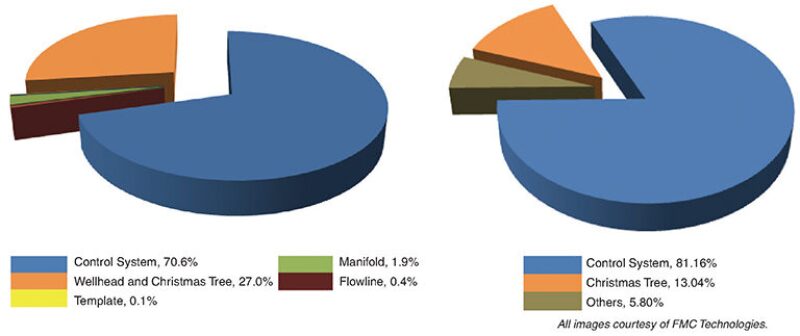
A closer look at control system failures shows that the subsea control module (SCM) is the largest contributor (33%) to subsystem failures. Further examination of the critical failure modes of the module (Fig. 2-above) reveals that the failure rate of the control/signal (58%) is the largest contributor to single well shutdown. The ability to monitor the condition of the SCM and plan ahead for maintenance is, therefore, crucial in reducing downtime.
Theoretical Model
A Markov chain is a method to study the transition from one state to another based on conditional probabilities (Fig. 3). It is commonly used to describe systems that follow a chain of linked events, in which what happens next depends on the current state of the system. The effect of introducing a condition monitoring system on the availability of equipment is demonstrated using this approach.
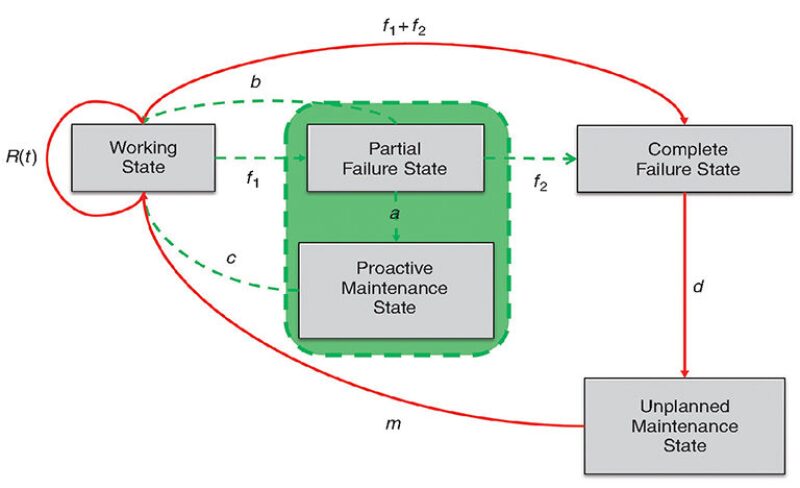
The key differences in modeling a system with condition monitoring and one without are:
- There is no complete loss in equipment functionality when the condition monitoring system proactively detects a failure. Most of the functions of the equipment are still available. This is represented by the restoration parameter b from the partial failure state to the working state as represented in the Markov chain. For the purpose of this analysis, b=0.80, representing that 80% equipment function is still available when condition monitoring system detects a potential failure.
- The probability of equipment failure is distributed such that the condition monitoring system will detect the failure before total equipment failure happens. This transfers the susceptibility of failure to the condition monitoring system and reduces the likelihood of equipment failure.
In this analysis, we define the failure transfer ratio as follows:
Failure transfer ratio=
Failure probability from working state to partial failure state
Failure probability from working state to complete failure state
=f1/(f1 + f2)
A higher ratio correlates to a greater effectiveness of the condition monitoring system to diagnose and detect failures. In this analysis, the failure transfer ratio is assumed to be 0.95, meaning that the condition monitoring system is capable of diagnosing 95% of failures on the equipment. Weibul distribution is used in this analysis.
The Value of Condition Monitoring
Condition monitoring provides real-time visibility on the condition of the system. Partial system failures are also readily detected and diagnosed, allowing forward planning for maintenance. Such maintenance is typically more cost-efficient than performing reactive maintenance, where the extent of failure and associated costs are far greater.
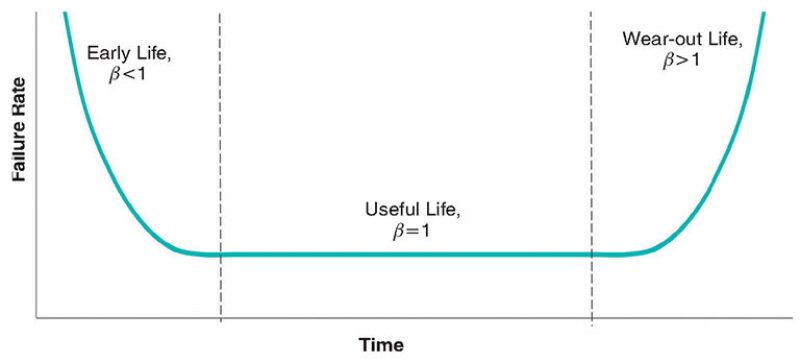
The life cycle of equipment can be represented by three regions of the bathtub curve, characterized by the β; value (Fig. 4). A particular analysis was performed for a β value of 0.5. This denotes the installation of the condition monitoring system in the equipment’s early life. The results indicate: a) Lower probability of maintenance for equipment with condition monitoring (Fig. 5); and b) Higher equipment availability with condition monitoring, up to 18.5%. This trend was found to be true for all regions of the bathtub curve.
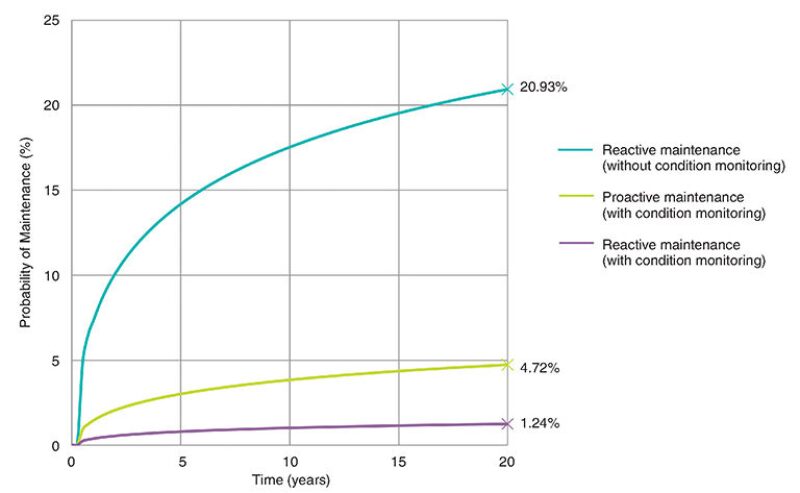
Operational Example
The first full condition monitoring system by FMC Technologies, Condition and Performance Monitoring (CPM), was delivered to an oil and gas field in the North Sea. The system consists of data collection software and historical data storing, together with an onshore analysis server.
The electronic components in the subsea router module are sealed at atmospheric pressure. An internal pressure transmitter records the pressure. Three weeks into the initial tuning of the CPM system, the system detected a continuous pressure increase. This prompted the operators to investigate further, and it was later concluded that the module’s internal pressure had been increasing for the past 2 months because of a weakness in the fiber penetrator on the subsea router module. The module continued operating for a further 3 months until failure, after which the subsea control system automatically switched to the redundant module.
The early detection allowed the operator to have full visibility of the module’s condition and subsequently prevented otherwise unforeseeable communication errors. Given early warning, the operator was provided sufficient time to concurrently plan an intervention campaign while troubleshooting the problem.
Conclusion
Subsea condition monitoring is one of the beneficial real-time diagnostic tools in the subsea production surveillance system. The benefits of the condition monitoring system were studied through the application of a Markov chain. The maintenance on equipment was distributed to two states representing proactive maintenance and reactive maintenance. The condition monitoring system was theoretically proven to increase the equipment’s availability, especially if the system is installed during the equipment’s early life. Although the analysis was performed at the equipment level, the resulting trend is the same when applied to a system with a high level of redundancy.
The more effective the condition monitoring system’s diagnosis, the higher the chance for it to capture the equipment degradation prior to failure and increase the equipment’s availability. For an efficient condition monitoring system, the type and amount of data received is critical to enable a comprehensive system of fault diagnosis. However, this remains a challenge as there is limitation on data access and availability from subsea operators.
Chen Yuru Serene is a reliability engineer for subsea engineering services at FMC Technologies, focusing on subsea reliability studies, facilitating the identification of potential risks, and running reliability, availability, and maintainability (RAM) analysis for subsea production systems. Serene holds a bachelor’s degree in mechanical engineering from Nanyang Technological University.
Leong Pei Chze is a life of field engineer at Forsys Subsea, focusing on subsea condition monitoring systems. Before this, she was a production performance services engineer and a controls and data management product design engineer at FMC Technologies. Pei Chze holds a bachelor’s degree in electrical and electronics engineering from Universiti Tenaga Nasional.